How Artificial Intelligence is advancing the energy industry
Bill Scudder, GM of AIoT Solutions at AspenTech talks about the significance of its cloud ready AIoT Hub
What is your AIoT Hub?
The Aspen AIoT (Artificial Intelligence of Things) Hub is a cloud-ready and built-for-purpose Industrial AI infrastructure that unlocks the immense value from data assets across capital-intensive enterprises to deliver actionable insights quickly and easily. The AIoT Hub’s powerful technology helps industrial organizations address today’s stringent requirements for safety, efficiency, and profitability, while helping to reduce waste and meet sustainability goals. Beyond supporting organizations’ current needs, the AIoT Hub is laying the foundation for a future in which technology enables self-optimising plants.
The Hub consists of several well established and innovative technologies. At its core is Aspen InfoPlus.21 (IP.21), the information management system for real-time industrial processes, which collects large volumes of process data for reporting and analysis. It also integrates technologies from several acquisitions we have made in recent years, including cloud native connectivity from RtTech Software, cloud capabilities and the ability to assemble and deploy AI-driven IoT applications from Mnubo, and enterprise insights and visualisation features from Sabisu.
How will you be rolling this out?
Lacking a complete solution, some vendors are attempting to cobble together AI, software, and domain knowledge from three or more disparate providers to meet the market needs. Aside from creating disjointed commercial arrangements, those efforts are falling short for customers, yielding lengthy projects that take an army of data scientists to implement and providing little more added value than piping together generic AI processes. Success requires a different approach.
Unlike generic AI platforms, which require a massive data integration effort and an army of data scientists to implement, the AIoT Hub can seamlessly integrate industrial data sources and deliver actionable insights faster than ever before with ready-to-use and built-for-industry applications. It is also extensible, enabling customers and partners to collaborate on Industrial AI models and deliver enriched applications.
How does the launch enable the move to Industry 4.0?
Over time, our vision is that the solutions we provide for asset optimization, performance engineering, production optimization, and in the reliability and maintenance area will support ever-increasing levels of automation, enabling more autonomous operations and the smart plant of the future.
The AIoT Hub enables seamless, flexible data mobility and integration across the plant, from sensors to the edge and cloud, and empowers organizations to unlock the value of their data faster than ever leveraging next-generation technology. It also allows data scientists and business analysts to collaborate and build their own data-rich AI models using the Aspen Industrial AI Toolkit.
By leveraging AspenTech’s existing portfolio of applications, the AIoT Hub provides a holistic understanding of data for business intelligence across the enterprise, with data visualisation, historical contextualisation, analysis and more. Overall, the AIOT Hub addresses a key challenge for many industrial organisations today: the lack of a scalable data infrastructure to power Industrial AI models from training to productisation. Hundreds of cases can be run in minutes to determine the best ways to increase margin.
What are the benefits of industrial AI?
Efficiency and value capture is everything in this economy. With the supply chain as fragile as it is, manufacturers and industrial companies cannot risk errors and machine failures slowing them down. Predictive and prescriptive maintenance technology with machine learning and Industrial AI can identify precise failure patterns to predict equipment degradation weeks or even months in advance so action can be taken to avoid unplanned downtime and associated safety and environmental risks.
Additionally, Industrial AI, when implemented successfully, unlocks new opportunities for capital-intensive organisations looking to unify their industrial AI and sustainability strategies to create a better plant of the future — by enabling safer, greener, longer, and faster operations.
As more companies begin implementing specific process metrics that consider emissions and resource use, organizations are increasingly focusing on waste and discharge reduction from production units, as well as efficiency enhancements through digital technology. The International Energy Agency (IEA) has found that Industrial AI and digital solutions can help boost energy efficiency as much as 30% for industrial operations. Multi-dimensional optimisation, predictive analytics, and other digital solutions will provide the visibility, mobility, analysis, and insight needed to address the challenges inherent in meeting sustainability goals.
What are the challenges you face in terms of AI adoption?
In recent years, there has been considerable investment in democratising access to AI technologies through various AI/machine learning (ML) platforms, frameworks, and toolkits. But while this has accelerated the enablement of AI-based use cases, it has not necessarily translated to significant business value, especially within the industrial sector. According to the AI: Built to Scale study by Accenture, nearly 69 percent of executives acknowledge they know how to pilot a program, but they struggle to scale their Industrial AI strategy across the enterprise.
While exploring and identifying Industrial AI-enabled use cases may be intriguing, the starting point of any organisational strategy is never the technology. It begins with identifying the business problems, corporate objectives, and strategic goals. To overcome this adoption hurdle, organisations within capital-intensive industries need to place increased emphasis on democratising the application of AI to domain-specific industrial challenges with a focus on business outcomes. The key to making Industrial AI work in real-world applications is getting the learning right — and more importantly, making it valuable and actionable in an industrial business context. Therefore, the development of Industrial AI-enabled applications needs to be purposefully guided by domain knowledge to derive real business value.
How is industrial AI being used in the energy sector?
We are seeing investment in areas like remote plant operations and maintenance, as well as supply chain visibility.
For example, Saras, a leading independent energy and refining player based in Europe, established a Digital Predictive Maintenance Center that unifies all data so reliability engineers can rapidly assess and correct reliability issues. Saras wanted to move from reactive to proactive maintenance, taking a data-driven approach where predictive analytics define maintenance needs. To accomplish this, they selected and deployed Aspen Mtell on over 50 large assets in a refinery and are adding assets at a wind farm to reduce turbine maintenance costs. By implementing this, they were able to reduce unplanned downtime, avoid millions of euros in lost production opportunity, reduce maintenance costs, and detect 5 turbine gearbox failures in the wind farm with no false positives.
KEEPING THE ENERGY INDUSTRY CONNECTED
Subscribe to our newsletter and get the best of Energy Connects directly to your inbox each week.
By subscribing, you agree to the processing of your personal data by dmg events as described in the Privacy Policy.
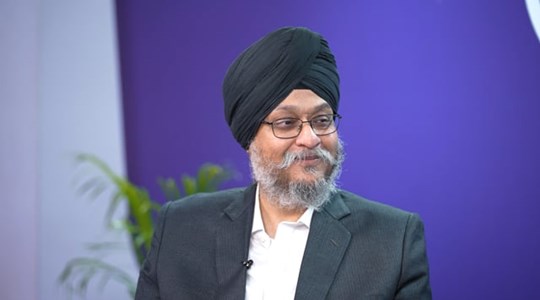
Why the energy industry is on the cusp of disruptive reinvention
Mar 12, 2024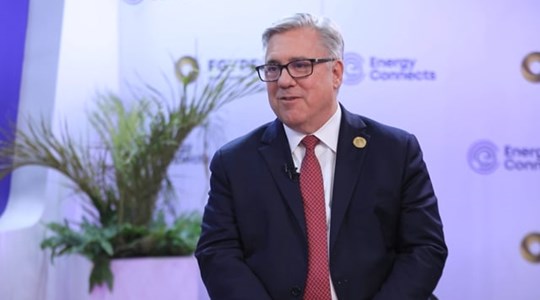
Chevron helping drive Egypt’s journey to become Africa’s energy powerhouse
Mar 11, 2024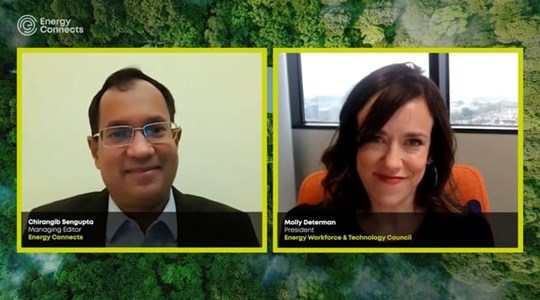
Energy Workforce helps bridge the gender gap in the industry
Mar 08, 2024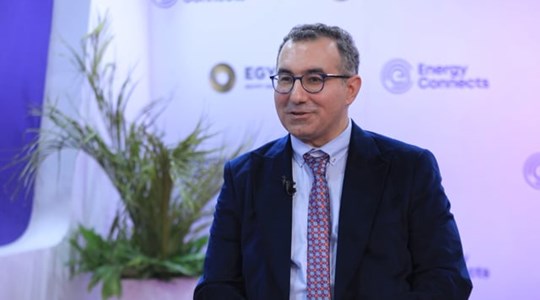
EGYPES Climatech champion on a mission to combat climate change
Mar 04, 2024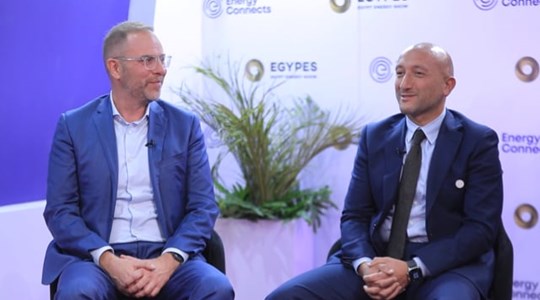
Fertiglobe’s sustainability journey
Feb 29, 2024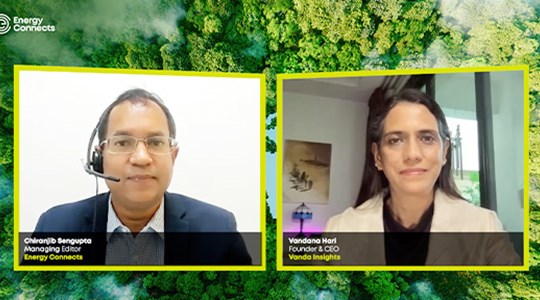
India’s energy sector presents lucrative opportunities for global companies
Jan 31, 2024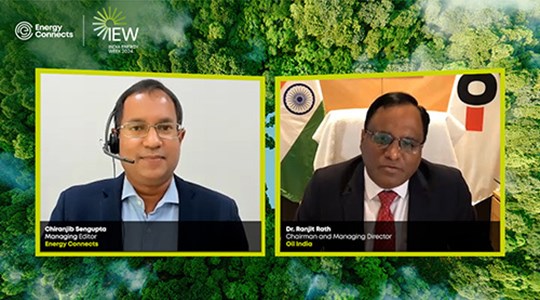
Oil India charts the course to ambitious energy growth
Jan 25, 2024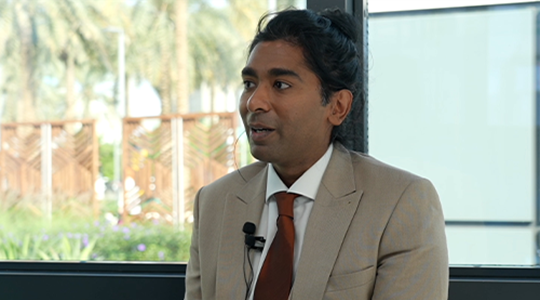
Maritime sector is stepping up to the challenges of decarbonisation
Jan 08, 2024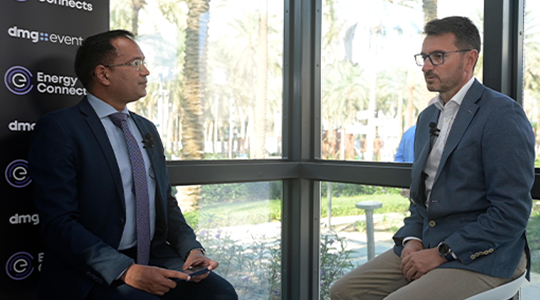
COP28: turning transition challenges into clean energy opportunities
Dec 08, 2023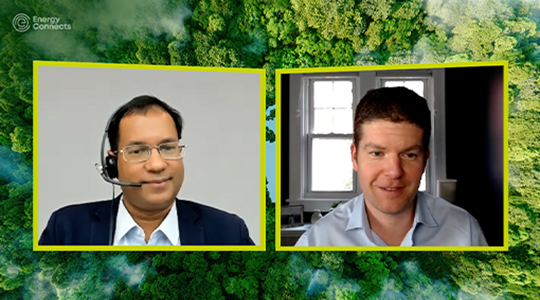
Why 2030 is a pivotal year in the race to net zero
Oct 26, 2023Partner content
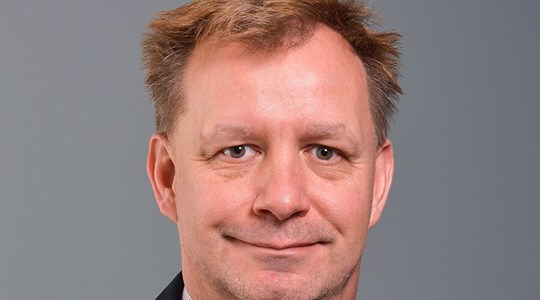
Ebara Elliott Energy offers a range of products for a sustainable energy economy
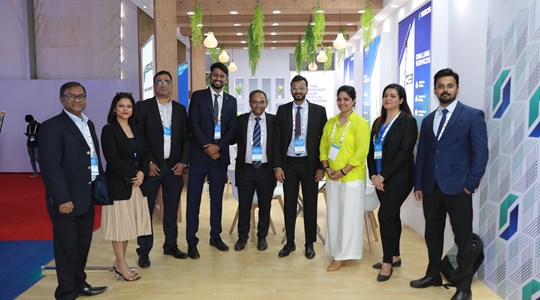
Essar outlines how its CBM contribution is bolstering for India’s energy landscape
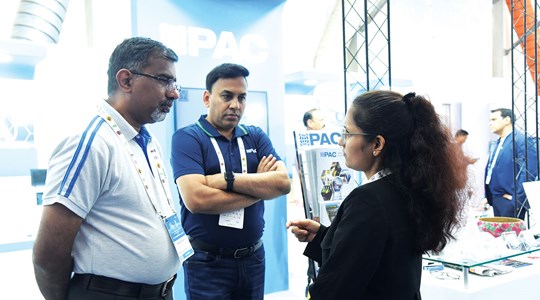
Positioning petrochemicals market in the emerging circular economy
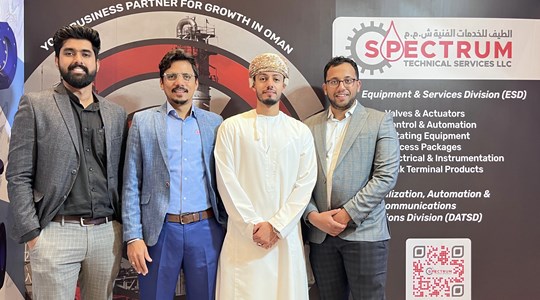
Navigating markets and creating significant regional opportunities with Spectrum
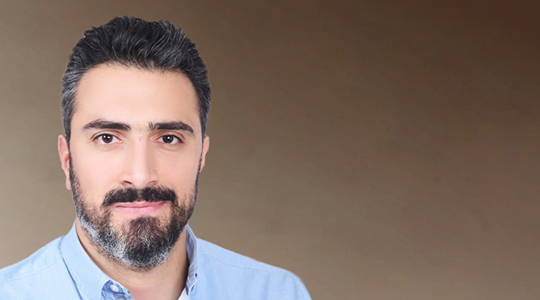